Take a look below. This is a cohort chart—one of the key charts in your Analytics account. If, like a typical marketing manager, you only have a few hours weekly to look into analytics reports, reviewing this chart is a must. It’s one of the very few charts in Google Analytics that directly indicate how retentive your marketing engine is.
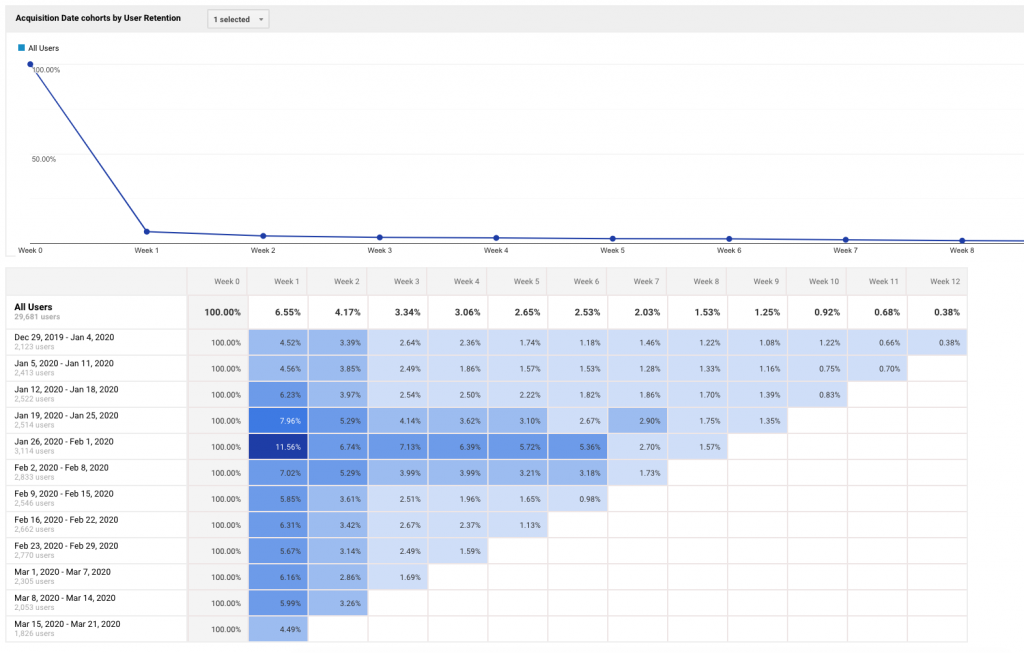
What makes this chart so important?
Most Analytics charts describe only individual components of your marketing. Sessions. Time on site. Conversions. Transactions. Viewed in isolation, these are vanity metrics. It’s very hard to understand all the interdependencies between such metrics and be confident about the impact of their deltas on key business metrics that marketing managers need to perfect (like sales). Also, none of them tell you anything about the quality of the overall online experience of your users. How sticky the experience is and how this stickiness changes over time. How much gravity your message, brand or marketing campaigns have. How often people come back for more. Are you acquiring more or less engaged users over time. When do users start churning etc…
In marketing, the stickiness of the experience is the only thing that matters. It’s only by looking at metrics in the life-time context—composed on natural patterns and cycles of usage—that one can derive actionable insights.
Fortunately, there’s one report that tells you more about that stickiness: cohort analysis report. If you have a very limited time to look into your Analytics, this report should definitely make it into the list of key charts.
In this article, I’ll walk you through the structure of this report and help you understand it, show what kind of insights you can derive, and how to extend it.
Fire up your Analytics and follow me!
Understanding cohort analysis
To launch your cohort report, simply go to the Audience section of your Analytics reports and click Cohort Analysis.
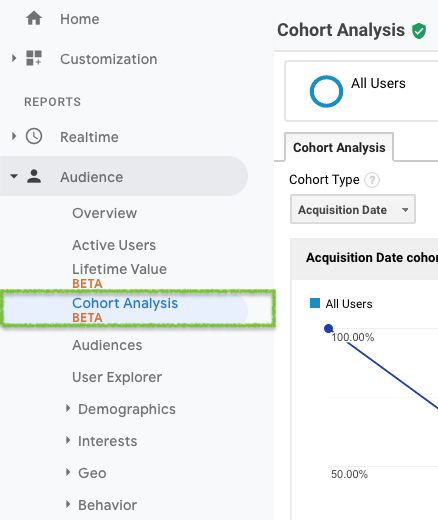
What’s cohort? Cohort simply means a group of users who share some characteristic or experience. It can be a date when they first were acquired, age, sex or city.
The point of cohort analysis—as Bounteous points out—is looking at these groups of people, over time, and seeing how their behaviour differs.
Cohort analysis is a relatively complex report with lots of moving parts, so let’s walk through it step by step. Top section first.
Top section first.

Cohort type is that shared characteristic we use to build cohorts. Currently the only option available in Analytics is the acquisition date. So our cohorts will essentially be groups of users who share the specific time window of acquisition. You can group users by days, weeks, or months. In Analytics terms, this time frame is called Cohort size.
The Metric is what we measure for each cohort. You can choose from 14 metrics of various types: per-user metrics, retention metrics, total (aggregate) metrics. An example of metric is user retention, defined as the number of users who returned to your website again in the Nth time period (day, week, month), divided by the total number of users in the cohort. Date range is the next chart parameter. It sets the time window for the report.
For example, tracking user retention by weekly cohorts (set up by acquisition date) over 12 weeks would help us understand how many users from each cohort (acquired in some specific week) were coming back to the website in the next weeks after acquisition. We would be able to see what was the retention rate of the users acquired in, say, first week of January, in as far as the second week of March.
Before we dive deeper into Analytics, first let’s take a look at the Intercom’s illustration on how to read cohort charts.
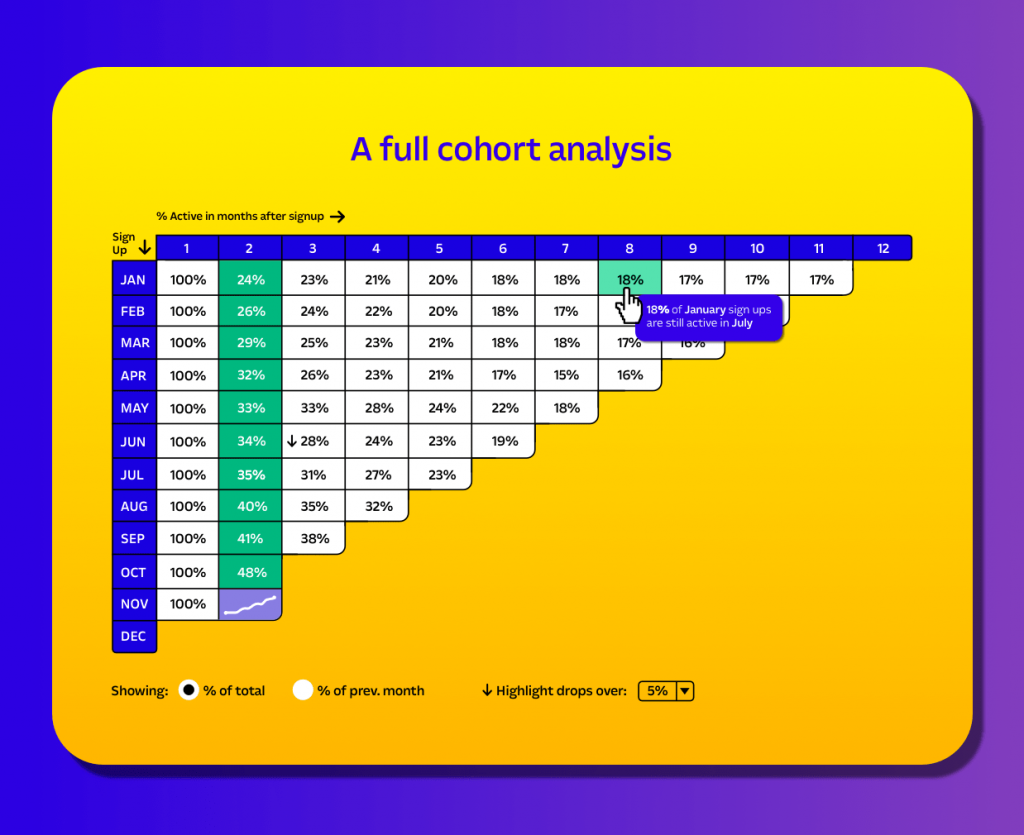
Below you’ll see a cohort graph produced by Analytics.
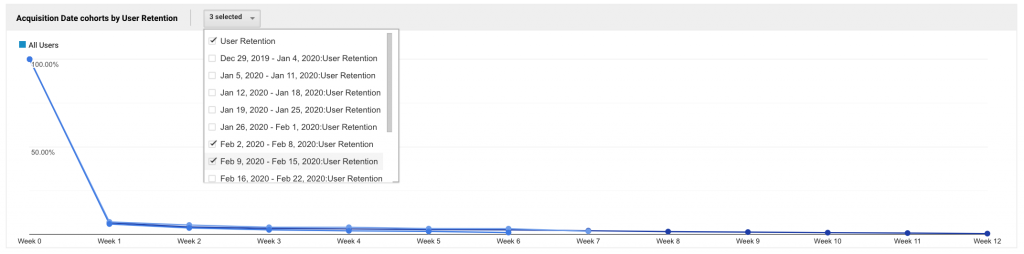
The graph section shows you the performance of the metric for all cohorts over specified date range. Vertical axis is metric value. Horizontal axis are the time period since the start of our measurement window. This graph works best for detecting outlier cohorts.
The sheer drop in metric value from time period 0 to 1, followed by much slower decay over the next period is common in such charts.
Below the graph you’ll see cohort table.

The first column shows cohorts and their user bases. The oldest cohorts on top. Be careful about cohort user base and always make sure it’s big enough to make relevant claims based on data. Rows are metric values for consecutive time periods since the start. Inside the cells, you’ll see the values for the metric of our choice for a given cohort and time after the start of measurement. In our example, this chart shows user retention values. Cohort tables have a triangle shape, because some cohorts haven’t been followed for the full date range (in our case 12 weeks).
The first row of the table (All Users) prints the average of the metric values of all cohorts for a given week, weighted by user bases. Default cohort graph you saw a second ago is built from values of this row. In our example, week-1 average user retention of 6.55% means that of all users who were acquired over the last 12 weeks (no matter which cohort they belong to), the average rate of return visit in the first week post acquisition is 6.55%. On average this many users visited the site again one week after being acquired regardless of the cohort. Similarly, 7-week user retention rate for all cohorts means that out of cohorts that were active then (had a nonzero user base for that week), on average 2.03% of users acquired in the first week were retained in the 7th week.
Data in the remaining rows takes into account users acquired in one specific week (or day or month depending on the cohort size you choose at the beginning) only. For example, in the week of January 26 – February 1 2020, there were 3114 users. For any other week of this cohort, the metric value you see in the cohort table is just the number of users retained in that week, divided by all the users that have been acquired in the week 0.
Currently, there’s no way to run cohort analysis for arbitrary dates in Analytics, and the longest time window is 3 months (either by running weekly cohorts for the date range of 12 weeks or monthly cohorts for the date range of 3 months).
The last feature we haven’t discussed yet is the colour heatmap for metric values. It can help you quickly grasp the decay over time. Significant differences in colour shade between columns is probably the fastest way to notice problems in the customer lifecycle between time periods. Nickelled says that by default, Google Analytics uses five different colours.
The last cell of each cohort is the current time period, so it’s typically less relevant.
Interpreting cohort chart
Broad perspective first. As I said, in my opinion cohort chart is one of the most important charts Google Analytics account. While most charts and graphs present a static perspective on your business – disconnected snapshots of performance of its various components like landing pages, blog sites or bounce rate, cohort chart says how well it’s working in making people visit and engage with your site back again to consume more of what you have to offer, be it content, e-commerce products or SaaS features. I believe that cohort chart offers a 3d image of your business.
Now let’s take some specific use cases. So far we’ve run cohort analysis on the user retention metric. It’s good to see how many people were coming back to your site in aggregate, but doesn’t tell anything about how engaged they were in aggregate terms, not to mention how engaged individual users were.
To get some additional intel on engagement, the following metrics should be useful:
- pageviews,
- session duration,
- sessions,
- users.
If you want to check engagement per individual user, it’s good to one of the per-capita metrics like:
- goal completions per user,
- pageviews per user,
- session duration per user,
- sessions per user.
It seems that the best cohort size for this group of metrics is week if you run a content-based website and days if you have a usage-heavy product such as subscription SaaS product. For e-commerce businesses, the best cohort size is month.
Running such reports with good parameters of cohort size and cohort time frame should do a great job of helping you understand how your marketing is performing in driving loyalty.
Let’s use cohort analysis to learn more about changes in engagement over time. Let’s run our analysis for weekly cohort sizes over 9 weeks for goal completions per user. Here’s what we get.
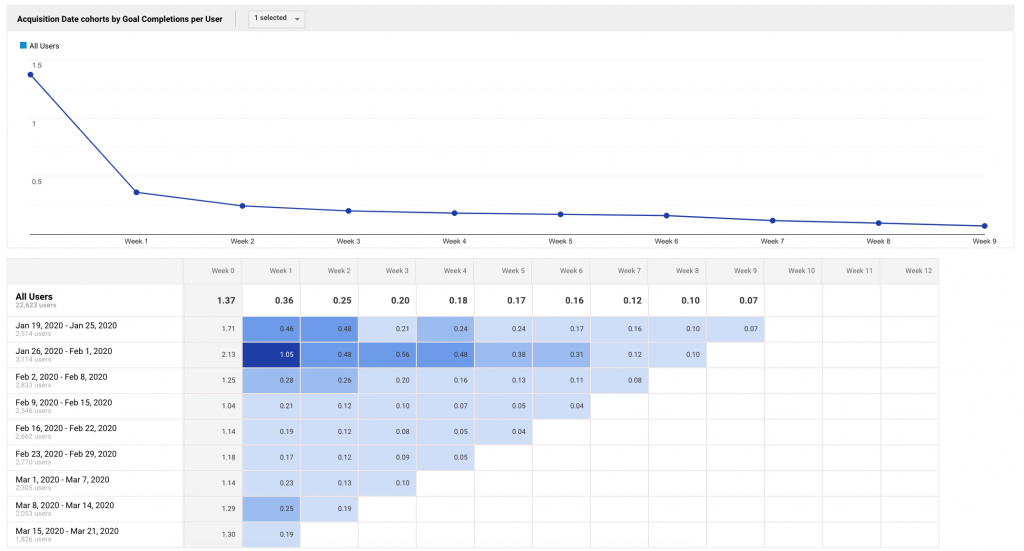
Clearly we see that there’s a problem in making people engaged around the content outside of the first week. We also see that the cohort acquired in the week of January 26 – February 1 had the highest metric value. To understand why, we’d need to dive deeper into our marketing actions of that week and try to find correlations in the content or tactics. Another way to try to understand this difference is to use additional segmentation and see what kind of marketing activities, city, device or other variables caused this anomaly. We’ll do this in the next part of this article.
Let’s now take a look at the aggregate session duration with the same cohort parameters as previously. Here’s what we get.
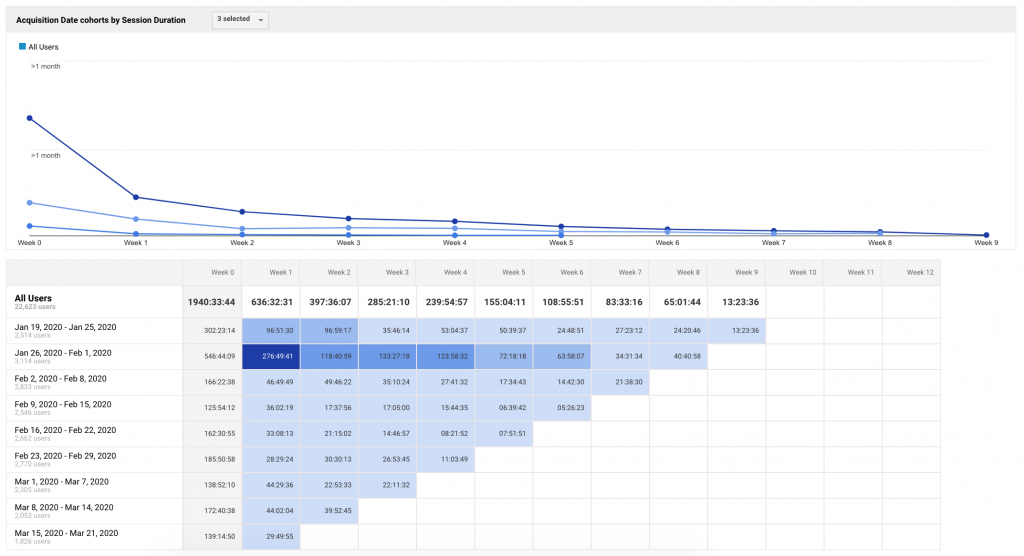
As you can see, we’ve also added two additional plots representing two individual cohorts, January 26 – February 1 and February 16 – February 22. Again, we see that there was an unusual engagement for the Jan 26 – Feb 1 cohort.
We can also use cohort analysis to learn more about the ability of the website or product to generate revenues, again both in aggregate as well as per user terms.
From all available metrics, metrics that will help to answer this question at best in aggregate terms are:
- transactions,
- revenue,
and on per user basis:
- transactions per user,
- revenue per user.
The best cohort size for this group of metrics is month as there are not many business where return purchases would be done on a daily or weekly basis. Revenue per user is particularly important in this case, as it maps directly to profitability and can tell you more about the amount of money you can spend on average to acquire one click or landing page visit or unique user. It can also help you understand how week-specific marketing activities on your blog or social media impact the revenue per user and hence can give you more intel about what to do in the future.

This chart depicts the amount of per capita revenue up to 2 months after the acquisition. This is one of the best charts to assess whether performance marketing activities provide a good return on investment. For example, in our analysis we had the average revenue of PLN6.33 in the first month following the month of acquisition.
This report can also shed the light the decay of sales. In our case, it can take up to two months for your marketing to generate the full sales effect, as clearly both for the December as well as January cohorts, the first months after acquisition still had quite strong per-revenue effects for your company.
It is also pretty clear that the second month after acquisition shouldn’t be expected to provide any additional return and can be useful in the content of choosing an appropriate remarketing groups. In this case, you should try using up to 45 days of the remarketing period to fully discount the sales effect assuming no change in marketing materials (e.g. website).
Of course these are all only potential ways to interpret the data not definite laws – every single claim here would have to be tested further before you could actually apply it to your business. Clearly cohort chart gives you a lot ways to interpret the data.
Cohorts as segments
Now let’s explore some additional features and options we can use with the cohort report.
First of all, you can combine cohort charts with custom segments to see how sticky is your marketing across other dimensions.
First, click Add segment.
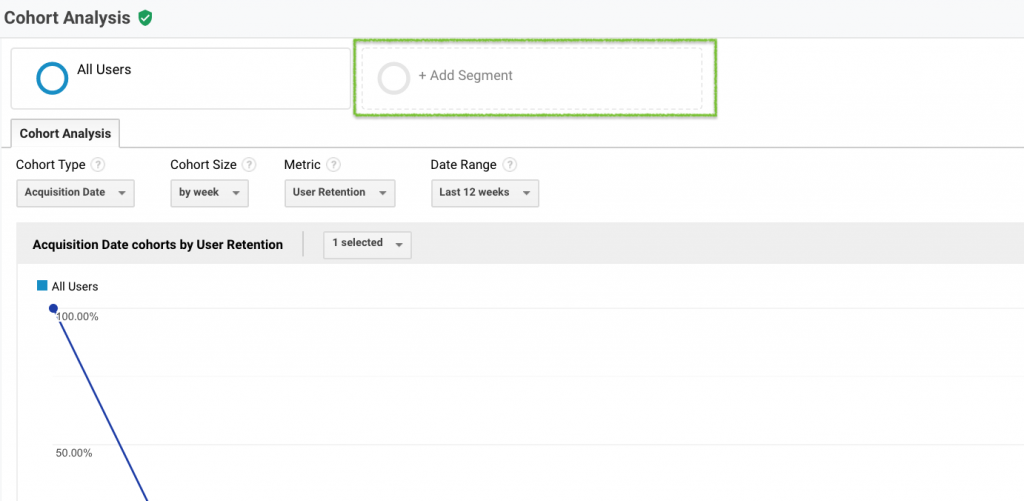
Click New segment.
Create segment conditions. In our example, so we’ll build segments for our key marketing channels. For starters, we’ll create the organic segment. For our metric, we’ll use the Goal Completions per User metric. Therefore, click Conditions, and make Default Channel Grouping dimension contain Organic Search. Save. See the new cohort table and new colour.
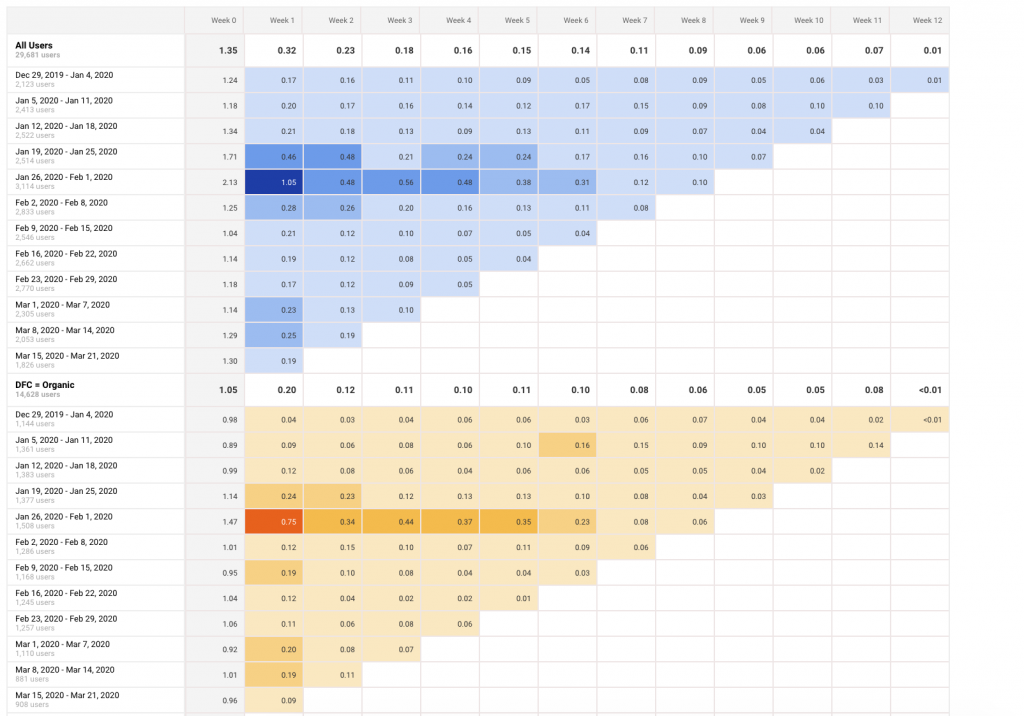
In the free version of Google Analytics, you can add up to four segments. We’ll add 2 more: CPC and direct.
(By the way, the quick way to create new segments based on the similar logic is to click on a “see more” arrow on a segment name and click Copy.)
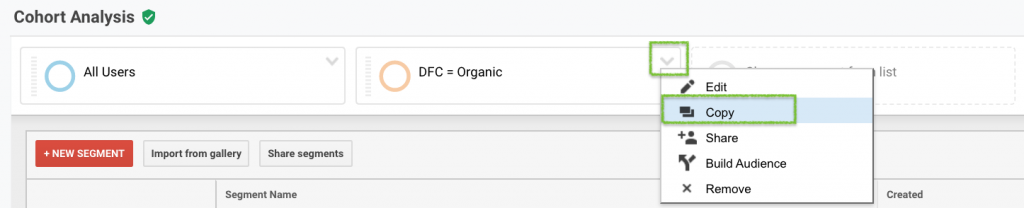
As a result, you’ll see the advanced segment panel again with default values from the source segment. In our case, we need to edit the Default Channel Grouping name to Paid Search. Do the same for Social. We now have 4 custom segments being applied to our cohort analysis.
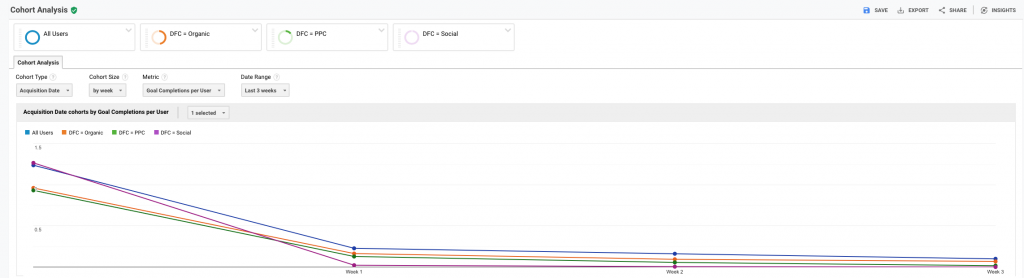
The results for the two new channels are as follows.
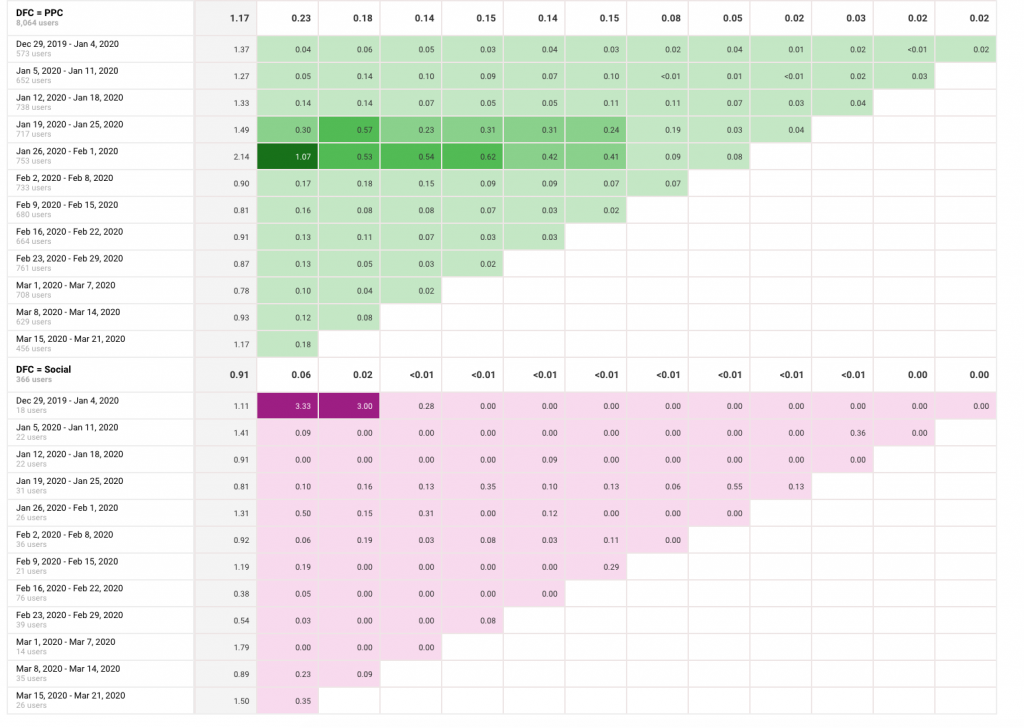
Based on these charts, we clearly see that there are some differences in how engaging various channels are. It seems like Social doesn’t typically generate much engagement, while organic and PPC are doing slightly better, but it also varies greatly among cohorts.
Also, we observe that no channel really generates long-term sticky experiences. There’s always a week where the engagement drops. If these reports were set up for a SaaS product with well-implemented goal conversions, it would mean that the product is not sticky enough and should be improved.
The second additional feature allows us to turn particular cohorts into new segments for further use in remarketing or other activities.
Let’s say we’re running our user retention analysis again on weekly cohorts with 12-week cohort window and want to re-activate one of the cohorts. We can do that easily with this feature.
All you have to do is to click on a cell you want to turn into a segment.
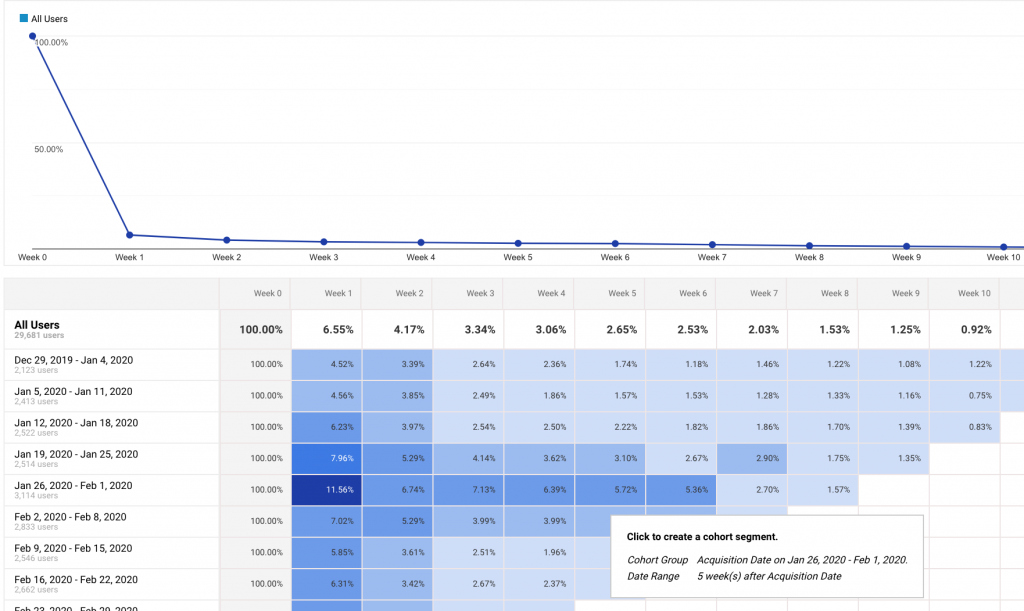
Once you click, you’ll see a popup window. Put down your segment name and voila!
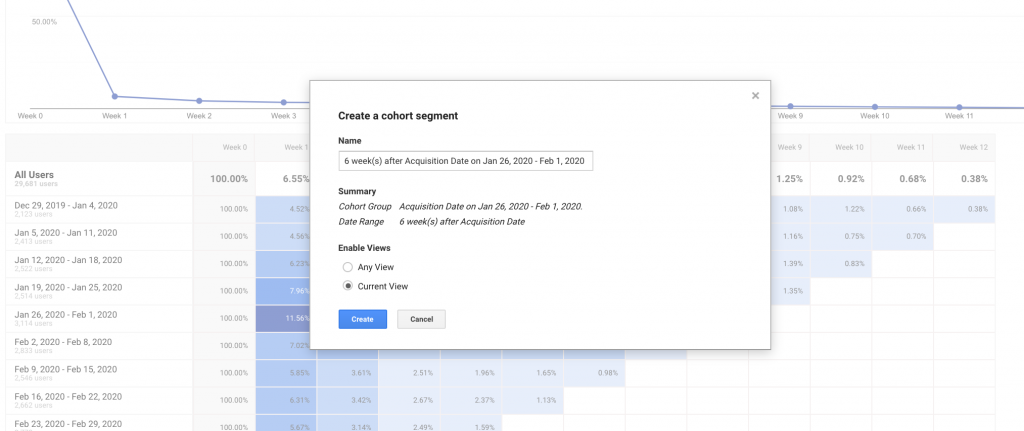
Click Create and this specific segment will be available for later use in your Analytics account. You can use it to create remarketing campaigns or analyse your account deeper.
Complications
There are a few things you need to keep in mind with this chart. As Bounteous points out, cohort analysis relies on how Analytics defined a user, which is just a unique set of cookies stored on a particular browser. Hence, subdomain and cross-domain tracking can confound data if you’re not using User ID feature.